Chemistry Professors Eugenia Kumacheva and Alán Aspuru-Guzik and their research groups are celebrating the publication of a major new article in Nature Communications.
“Self-driving lab for the photochemical synthesis of plasmonic nanoparticles with targeted structural and optical properties” describes the rollout of the Autonomous Fluidic Identification and Optimization Nanochemistry (AFION), a self-driving lab (SDL) that integrates a microfluidic reactor, in-flow spectroscopic nanoparticle characterization, and machine learning.
"The AFION lab was developed to identify or discover conditions for the photochemical synthesis of plasmonic nanoparticles with precisely targeted spectroscopic properties,” said Prof. Kumacheva.
Plasmonic nanoparticles (NPs), she explained, are used in solar cells, spectroscopy, imaging, in detecting tiny amounts of heavy metals, pesticides, pharmaceutical residues, pathogens and toxic gases, and in photothermal therapy for cancer treatment.
“By integrating machine learning with photochemical synthesis, we unlock new ways to efficiently explore complex reaction spaces in flow,” said Tianyi Wu, a graduate student in the Kumacheva lab and another of the authors of the study.
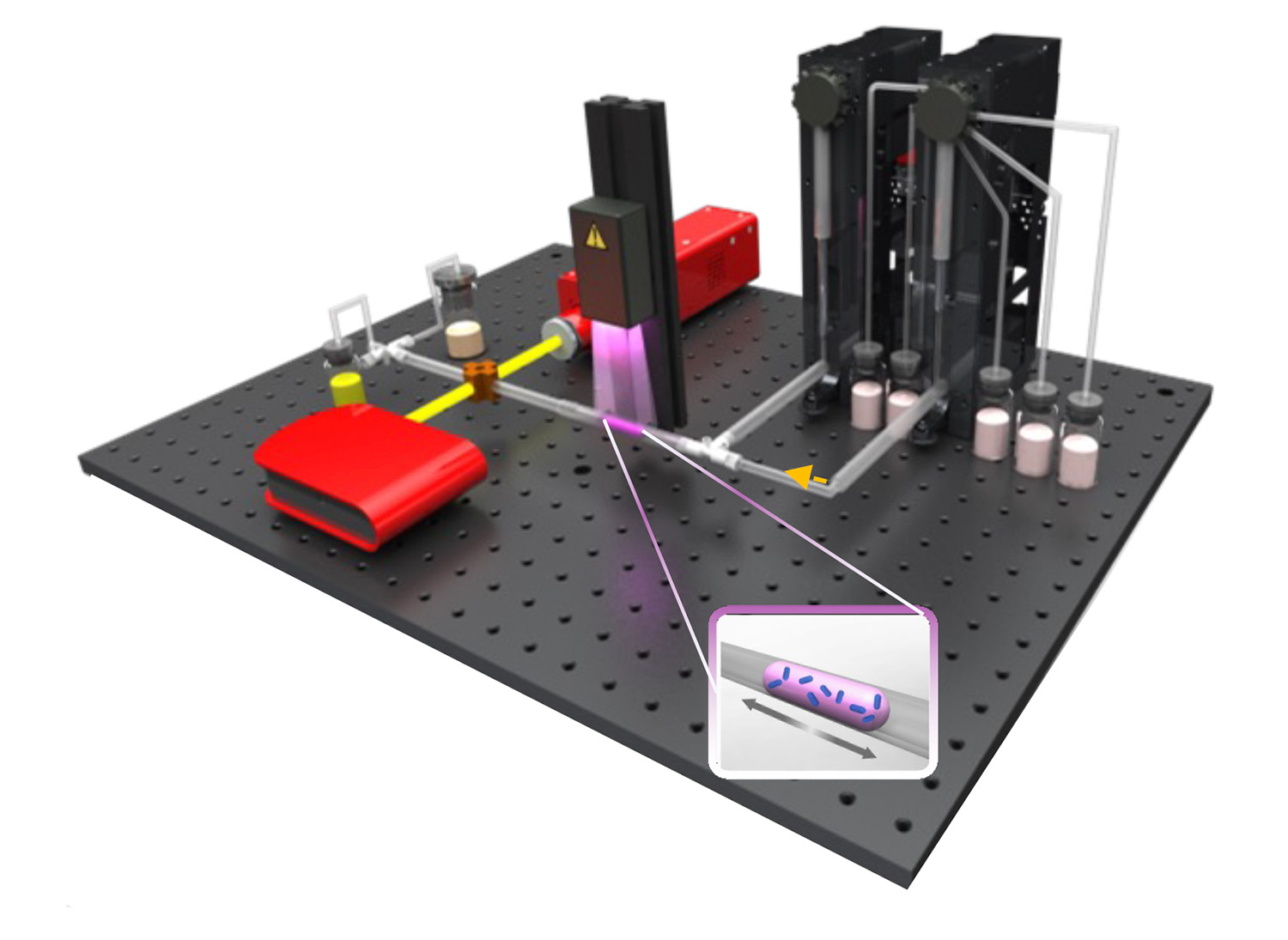
Wu took a leading role in developing and running the experiments on the AFION self-driving platform. “My contributions included building and programming the platform, defining synthesis goals, loading reagents, and validating the synthesized nanoparticles—such as imaging their sizes and shapes.”
“Since AFION is an automated system, it is programmed to autonomously run reactions and collect real-time feedback without human intervention, such as manually mixing chemicals and measuring results.”
In nanoparticle synthesis, Wu explained, many factors influence an NP’s final size, shape, and properties. These include reaction time, light intensity, and chemical concentrations—each of which, in turn, determines the nanoparticles’ potential applications. “In this study, we simultaneously optimized seven different parameters (or "dimensions"), leading to over 10 million possible experimental condition combinations."
The power of self-driving labs is that they can effectively explore even an impossible-seeming number of possibilities. “Testing so many combinations would be extremely time-consuming, but with AFION, we can efficiently explore this vast space and identify the best synthesis conditions in just thirty hours.”
If this seems impossible, even with advanced tech, it is because AI and machine learning make it unnecessary for AFION to serially perform all ten million experiments. “A closed-loop fluidic SDL system doesn’t just run experiments blindly—it learns from previous results and refines the next set of experiments.”
“In our case, the system automatically adjusts reaction conditions based on real-time feedback, helping find optimal synthesis conditions. This intelligent decision-making is powered by a sophisticated algorithm developed by Prof. Aspuru-Guzik’s group,” Wu said.
The “fluidic” aspect within AFION, meanwhile, refers to how reactions occur in a liquid-based setup, where chemical solutions flow through small channels. In traditional nanoparticle synthesis, reactions often take place in a regular beaker or flask, in amounts exceeding 10 milliliters, where chemicals are mixed in bulk. This approach becomes inconsistent because of factors like uneven mixing, temperature differences, and variations in how reagents are added—all of which can affect the final product.
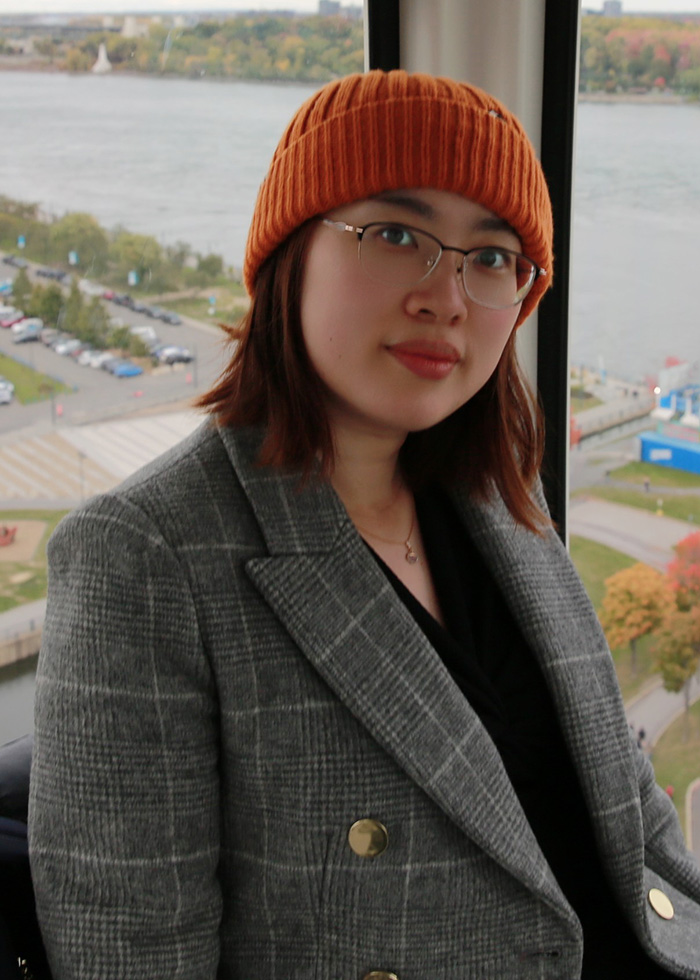
“It’s like baking a large cake,” Wu explained. “Heat may distribute unevenly, leading to some parts being overcooked while others are undercooked.”
AFION uses a fluidic system, where chemical solutions in tiny amounts (100 microliter) flow through narrow channels, providing a higher surface-to-volume ratio and better control over reagent interactions, leading to more uniform conditions and consistent results.
“It’s like baking many smaller cupcakes, each in its own compartment with controlled heating. Since each cupcake gets the same amount of heat and bakes under the same conditions, the results are much more consistent. Similarly, AFION ensures reactions happen under well-controlled conditions, reducing inconsistencies.”
“What excites me most is how this technology is revolutionizing nanoparticle synthesis,” said Wu. “Traditionally, optimizing or developing new materials involves a lot of trial and error, but AFION accelerates discovery by making smart, data-driven decisions. This allows us to explore a much wider range of materials and applications—from medicine to energy—in a fraction of the time and using significantly fewer resources.
Kumacheva agreed, emphasizing the significance of the research. “This is the first report of the closed-loop fluidic SDL that performs photochemical synthesis of eight types of diverse inorganic nanoparticles.”
“My estimate is that to achieve a similar target, an experienced scientist would need several weeks. "
Kumacheva is a member of the Acceleration Consortium, an organization where Aspuru-Guzik serves as the director, and whose mission is to champion the design and development of self-driving labs to accelerate the materials that we need the most. The work was also done in collaboration with Milad Abolhasani, a former student of Kumacheva’s who is now at North Carolina State University.
“Accelerating the discovery of new molecules and materials in this fashion, as well as developing green and sustainable ways to synthesize them, will help address global challenges in energy, sustainability and healthcare. All applications of plasmonic nanoparticles require precise control of their spectroscopic properties, and this is what we achieved... in an accelerated manner.”
AI and machine learning make it unnecessary for AFION to serially perform all ten million experiments. A closed-loop fluidic SDL system doesn’t just run experiments blindly—it learns from previous results and refines the next set of experiments. --Tianyi Wu